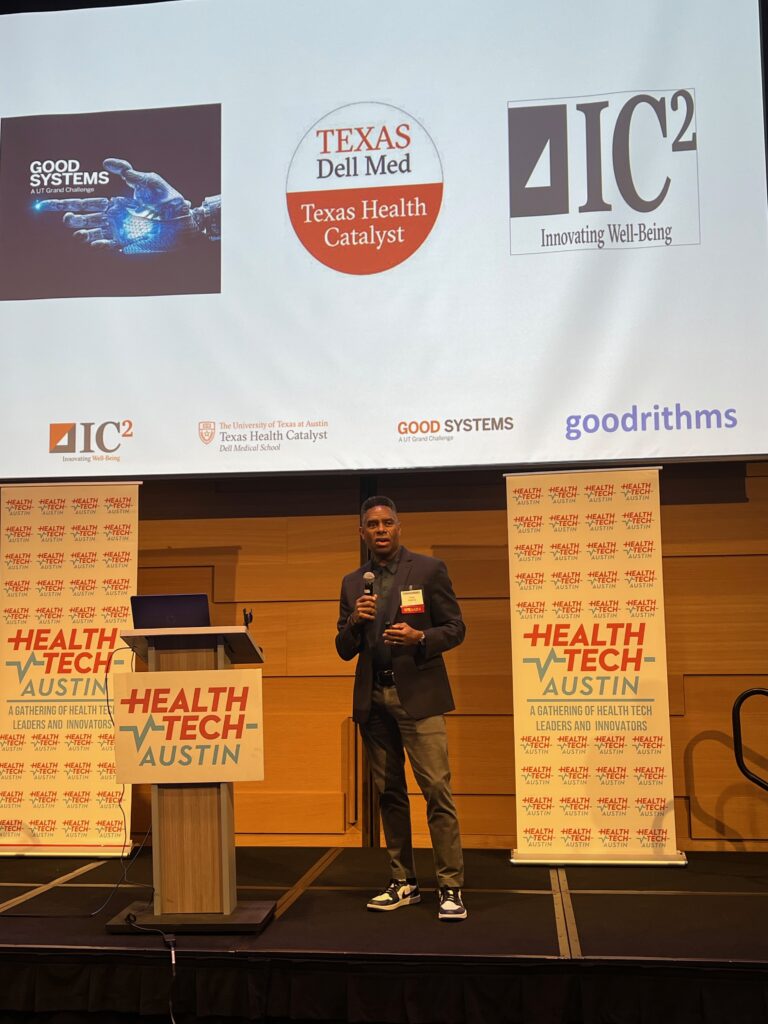
Incorrect algorithms in healthcare can mean life or death to patients.
Unfortunately, AI systems designed to help healthcare workers streamline patient care operations might exacerbate biases against people of color, according to Craig Watkins, executive director of IC2 Institute at UT-Austin.
Watkins spoke Tuesday morning at the Future of Health Summit, a half-day conference hosted by Health Tech Austin, at the Austin Public Library’s special events center downtown.
“We need to design systems and AI that are not intended to replace healthcare workers but to augment their work,” Watkins said.
Watkins is one of the principal investigators for UT Austin’s Good Systems Grand Challenge, which examines the social and ethical impacts of artificial intelligence. His research focuses on two core questions: How are bias and systems in inequities expressed in health artificial intelligence? How are researchers designing AI systems to address some of the system factors driving the behavioral health crisis in the U.S.?
Healthcare technology powered by AI needs to work in ways that don’t replicate the bias that exists in society, Watkins said.
Watkins cited a ProPublica investigative series from 2016 that looked at machine bias in software used by law enforcement to target criminals that are biased against Blacks. Watkins also cited a New York Times article that showed false facial recognition software incorrectly matched an eight-month pregnant Black woman to a carjacking.
“Facial recognition has a significantly higher error rate with people of color,” Watkins said.
AI systems are beginning to undermine healthcare, he said
Fixing racial bias in an essential COVID diagnostic tool that measures oxygen in the blood could have helped people of color during the COVID-19 pandemic receive better healthcare, Watkins. He said the diagnostic tool needed to be more accurate when measuring the oxygen level in the blood of people with darker skin.
In another case, Chat GPT-4 was significantly less likely to recommend advanced imaging (CT, MRI, or abdominal ultrasound) for Black patients when compared to their Caucasian counterparts, Watkins said.
And it takes work to correct the bias in existing AI systems, Watkins said. In an experiment using medical X-ray images, Watkins said AI algorithms could detect race even when all markers had been removed.
“Researchers still don’t know how these algorithms can predict race when they remove all markers,” Watkins said. “These models behave in ways we cannot fully understand or comprehend.”
At UT Austin, Watkins and his research team created an AI-powered chatbot to support parents dealing with postpartum depression. His team focused on training a system that could respond in high-stakes situations the way a healthcare provider might respond.
Watkin’s team is also spearheading an effort to design an AI-based chatbot to deal with mental health issues. His team is part of the Texas Health Catalyst program at the Dell Medical School. The focus is to reduce suicide and homicide rates among young people.
“The future of all this is evolving as we convene here today,” Watkins said. “How do you design systems that are inclusive?”
To address the issue further, UT Austin is hosting a conference on April 4 called “Health AI for All.”